AusIMM Melbourne Branch technical talk recap
The AusIMM Melbourne Branch recently hosted Dr Zeljka Pokrajcic for a technical talk on the application of orebody learning to meld geological information in the block model with downstream operational performance data for prediction and optimisation using machine learning.
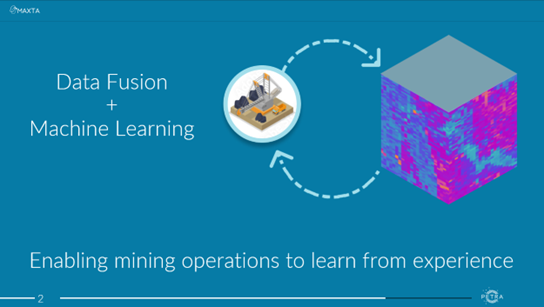
The orebody learning process in many instances uses existing operational data from disparate sources to merge all stages of the mining value chain using data fusion ore tracking. It enables operations to learn from previous operational experience, and leverages the rich repository of orebody knowledge sitting in the geology block model to provide new block model variables for downstream processes. This includes things like shovel dig-rate, plant throughput and recovery, as well as optimal blast designs and plant set points.
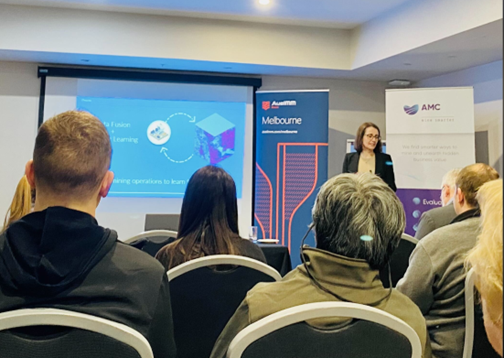
Dr Pokrajcic provided a brief background on machine learning and how it is now readily available, without the need to code, through freely available open source drag and drop software. Feedback from attendees showed the use of machine learning by geologists and engineers is happening now with many attendees applying the techniques in their everyday work.
A few case studies were presented showing the application of orebody learning at a number of operations. This included the identification of carbonaceous preg-robbing zones within the orebody, which historically reduced gold recovery to less than 50 per cent at an open-pit operation in Laos. The identification of problematic carbonaceous blocks allowed pre-emptive blending or allocation of these blocks to waste heaps.
A second case study showed how orebody learning was used in operational decision support. This knowledge provided operators of a large flotation circuit set point recommendations according to ore properties entering the plant, as well as prediction of flotation tail grade ahead of time. This helped improve operational stability and recovery.
Finally, Dr Pokrajcic outlined some of the perceived issues with data quality and reliability. Quoting Tim Napier Munn, “error is ubiquitous, we have to embrace and manage it with statistical techniques”, some of which are employed in orebody learning. This includes the calibration and autocorrection of on-line sensors, anomaly detection and real-time switching between machine learning inputs.
One of the key takeaways was how many different types of data can be used in orebody learning to predict, simulate and optimise operations using mechanisms to bring geological knowledge to downstream processes.
A diverse audience asked many questions about application of machine learning to all different aspects of operations. Great to see such engagement!